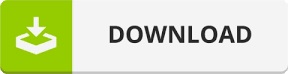

They save time and money and give everybody a chance to express themselves creatively. They change the way we think and talk about art as a whole.
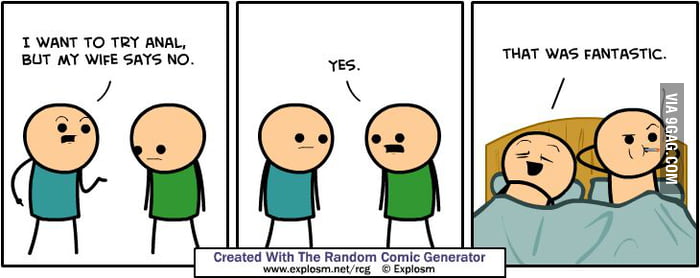
These AI image generators signal great achievement for humanity.
#Ai random meme generator full
While we may someday have full open access to these generators, right now, we’re limited in our ability to access and play around with these advanced technologies. Midjourney has a semi-open beta that requires a fee to join. StableDiffusion is open to the public for a fee, with a demo running on Hugging Face, a platform that hosts open source AI for public consumption. DALL-E 2 announced that they were opening up to the public on September 28th, 2022, after having a months-long waitlist that was only open to those working in tech. The current barrier with all AI image generators is that their technology is still developing so they are only available to the public in varying degrees. This guide is a breakdown of the best ways to use AI for memes. Some could be used to make memes while others are built with memes in mind. What AI is out there to help? And is it good for memes? Sure AI-generated images can be complex and human-like, but can they also be funny and hilarious memes? As AI technologies are released to the public, it should be clear what the best use for each one is. With advancements in AI Image Generation technology, the door is open for easier, and faster meme creation tools.
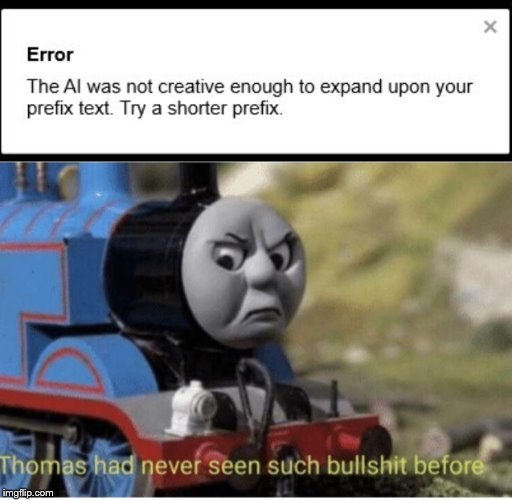
AI, however, could cut down on that time significantly. Even though they may look simple as a finished product, memes take time. Users then take the time to add text and stickers, making sure everything is correctly formatted and conveys the message they want to portray. Either in photoshop or on Instagram or using your favorite meme generator. Once the image is found, edits have to be made. This can take a while, even if you’re sure it's saved on your camera roll. When making a meme from scratch, you have to first find the right image. This procedure can also be seen as a kind of language-guided search, and can have a dramatic impact on sample quality.Without AI, the process of meme making can be long and complicated. Similar to the rejection sampling used in VQVAE-2, we use CLIP to rerank the top 32 of 512 samples for each caption in all of the interactive visuals. al explores sampling-based strategies for image generation that leverage pretrained multimodal discriminative models. Other work incorporates additional sources of supervision during training to improve image quality. This is interesting to compare to our reranking with CLIP, which is done offline. AttnGAN incorporates attention between the text and image features, and proposes a contrastive text-image feature matching loss as an auxiliary objective. StackGAN and StackGAN++ use multi-scale GANs to scale up the image resolution and improve visual fidelity. The embeddings are produced by an encoder pretrained using a contrastive loss, not unlike CLIP. al, whose approach uses a GAN conditioned on text embeddings.
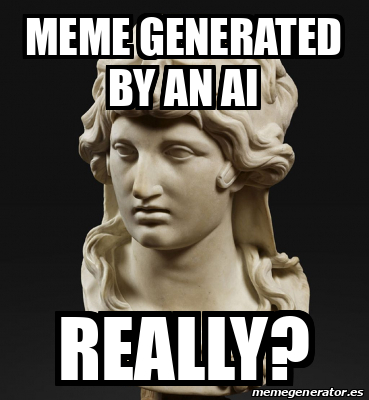
Text-to-image synthesis has been an active area of research since the pioneering work of Reed et. We provide more details about the architecture and training procedure in our paper. DALL♾ uses the standard causal mask for the text tokens, and sparse attention for the image tokens with either a row, column, or convolutional attention pattern, depending on the layer. The attention mask at each of its 64 self-attention layers allows each image token to attend to all text tokens. Finally, the transformations “a sketch of the animal” and “a cell phone case with the animal” explore the use of this capability for illustrations and product design.ĭALL♾ is a simple decoder-only transformer that receives both the text and the image as a single stream of 1280 tokens-256 for the text and 1024 for the image-and models all of them autoregressively. Those that only change the color of the animal, such as “animal colored pink,” are less reliable, but show that DALL♾ is sometimes capable of segmenting the animal from the background. Other transformations, such as “animal with sunglasses” and “animal wearing a bow tie,” require placing the accessory on the correct part of the animal’s body. This works less reliably, and for several of the photos, DALL♾ only generates plausible completions in one or two instances. The transformation “animal in extreme close-up view” requires DALL♾ to recognize the breed of the animal in the photo, and render it up close with the appropriate details. The most straightforward ones, such as “photo colored pink” and “photo reflected upside-down,” also tend to be the most reliable, although the photo is often not copied or reflected exactly. We find that DALL♾ is able to apply several kinds of image transformations to photos of animals, with varying degrees of reliability.
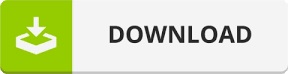